Recurrent Neural Networks (RNN): The Future of Predictive Text
4 min read
08 Jul 2024

Recurrent Neural Networks (RNNs) are at the forefront of natural language processing (NLP) technologies, driving advancements in predictive text and language modeling. This article explores the architecture of RNNs, their applications in predictive text and beyond, and the potential they hold for revolutionizing how we interact with written language.
Understanding Recurrent Neural Networks
RNNs are a class of artificial neural networks designed for sequential data processing, making them ideal for tasks involving time-series data or sequential dependencies. They utilize feedback loops to maintain information over time, allowing them to capture context and relationships between elements in a sequence.
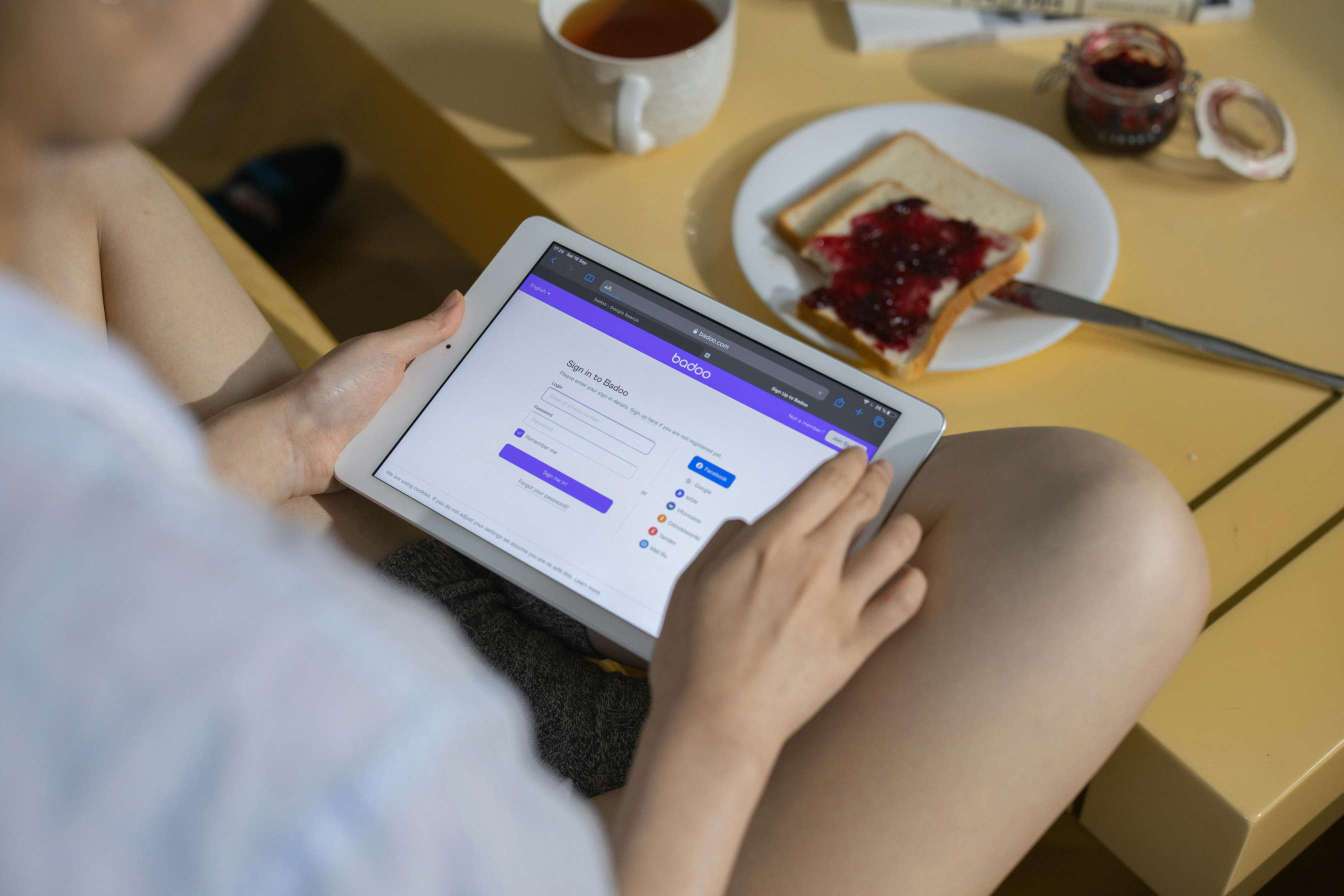
Applications of Recurrent Neural Networks
Predictive Text: Powering autocomplete features, next-word prediction, and text generation in messaging apps, search engines, and virtual assistants.
Speech Recognition: Enhancing speech-to-text systems by processing audio data in real-time and improving accuracy based on context and previous inputs.
Language Translation: Facilitating machine translation by understanding and generating sequences of words or phrases in different languages.
Financial Forecasting: Analyzing time-series data to predict stock prices, market trends, and economic indicators with higher accuracy and reliability.
Benefits of Recurrent Neural Networks
Sequential Learning: Excelling in tasks requiring memory and sequential dependencies, such as handwriting recognition and music composition.
Flexibility: Adapting to varying lengths of input sequences and handling dynamic patterns in data, making them suitable for diverse applications in NLP and beyond.
Real-Time Processing: Supporting real-time decision-making and interaction by processing incoming data streams continuously and efficiently.
Transfer Learning: Leveraging pre-trained RNN models and fine-tuning them for specific tasks, reducing training time and improving performance in new applications.
Challenges and Considerations
Vanishing and Exploding Gradient Problems: Addressing issues with gradient propagation in deep RNN architectures to maintain stable learning and improve convergence.
Long-Term Dependencies: Mitigating challenges in capturing and retaining information over long sequences, which can affect the accuracy of predictions and generation tasks.
Computational Complexity: Optimizing RNN architectures and algorithms to handle large datasets and reduce training time, especially for complex applications like speech recognition and language translation.
Future Directions
As RNNs evolve with advancements in deep learning, attention mechanisms, and memory-augmented networks, they are expected to drive innovations in NLP, predictive modeling, and intelligent data analysis. By enhancing predictive text capabilities and understanding contextual nuances in language, RNNs are paving the way for more intuitive and responsive AI systems that transform how we communicate and interact with technology.
More Articles
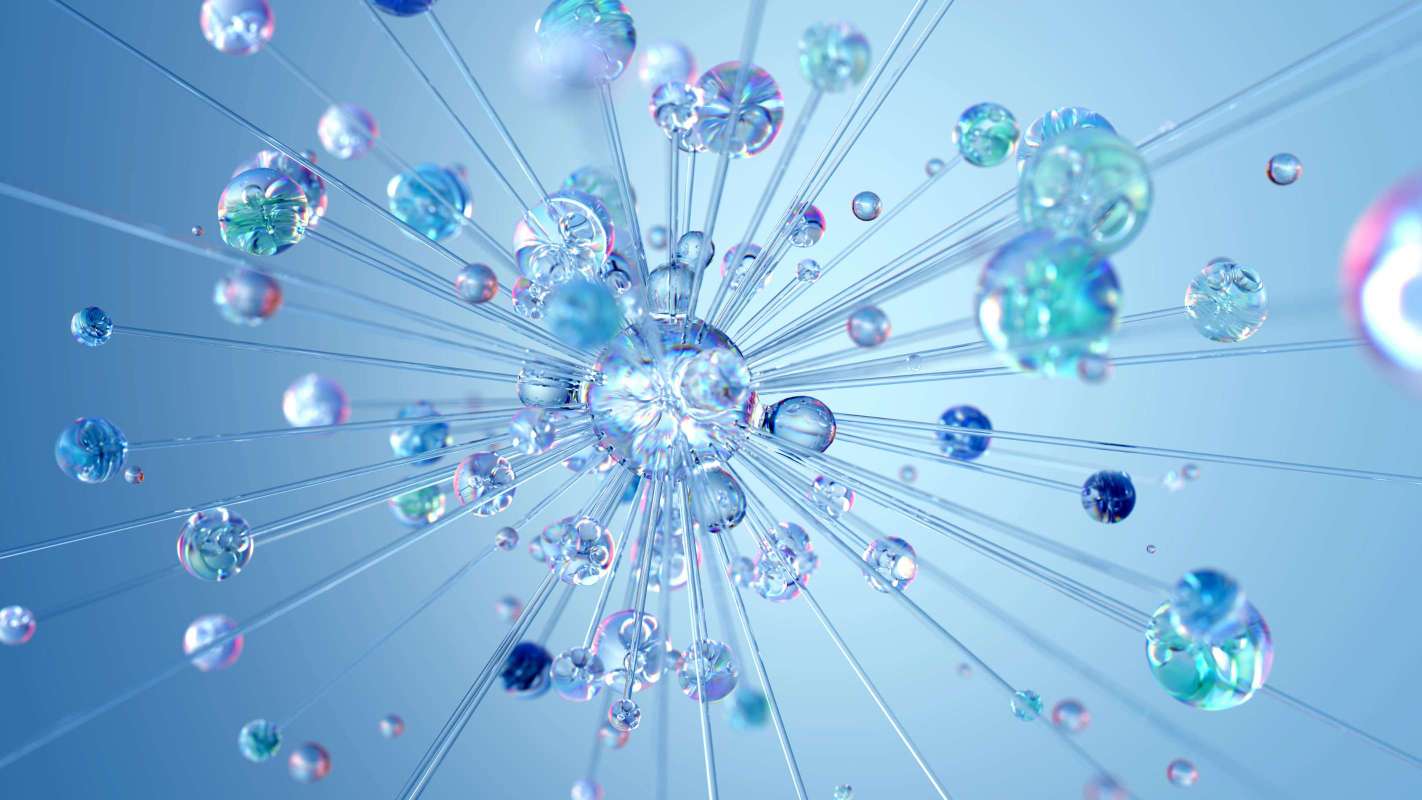
Artificial Neural Networks: The Technology That's Changing Everything
6 min read | 06 Jul 2024
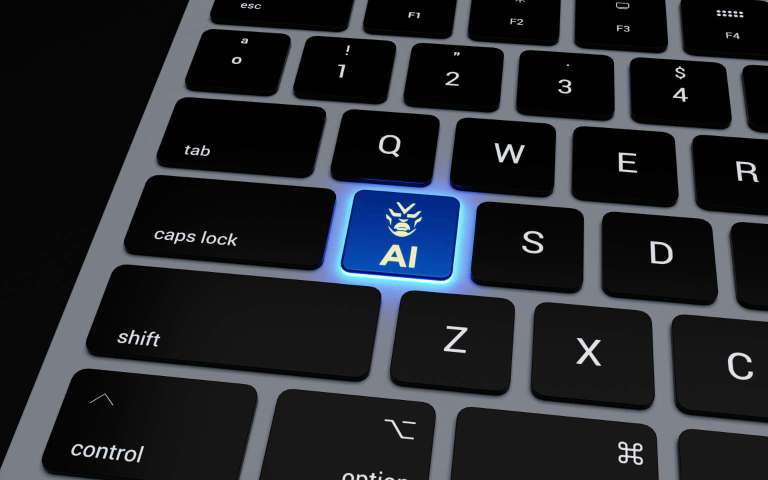
Cognitive Computing: The Next Big Thing in AI?
5 min read | 05 Jul 2024

Intelligent Automation: How Robots Are Taking Over Mundane Tasks
5 min read | 04 Jul 2024
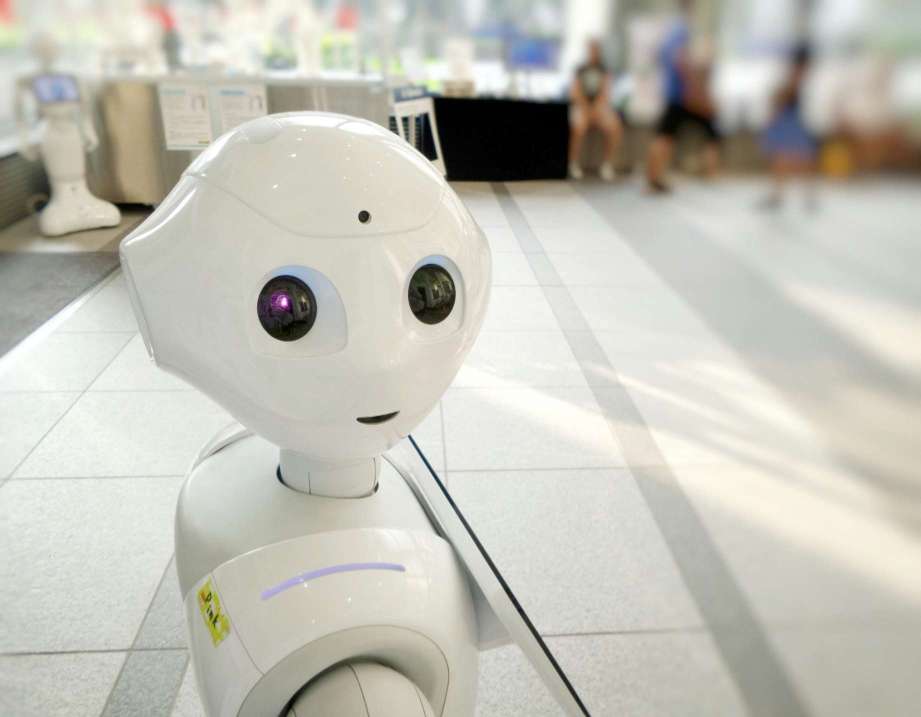
Robotic Process Automation (RPA) – Is Your Job at Risk?
7 min read | 03 Jul 2024
More Articles

AI Odyssey: Journeying through the Realm of Machine Learning
5 min read | 17 Jul 2024

The Quantum Leap: AI & ML Redefining Possibilities
5 min read | 16 Jul 2024

Data Symphony: Harmonizing AI and ML for Innovation
6 min read | 15 Jul 2024
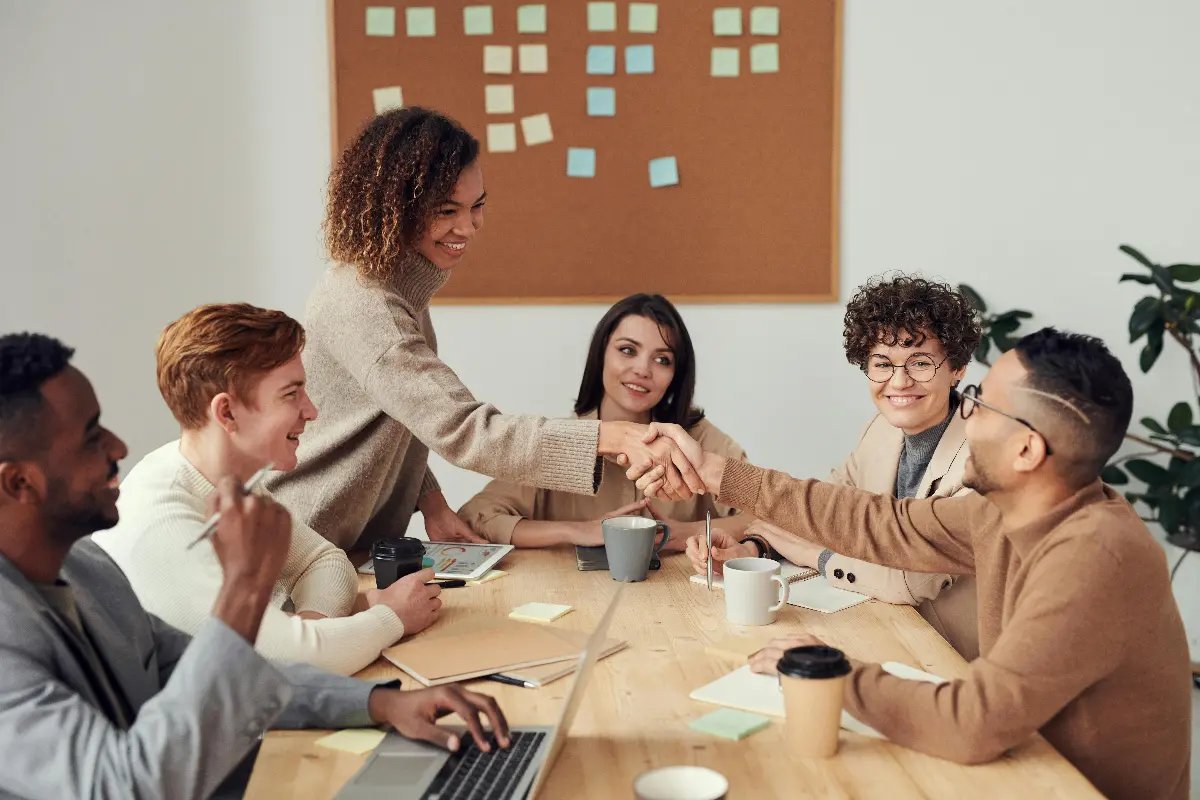
AI Governance: Policies and Regulations for AI Development
2 min read | 14 Jul 2024