Natural Language Processing (NLP) and its Impact on AI
4 min read
01 Jul 2024

Natural Language Processing (NLP) is a branch of artificial intelligence (AI) that focuses on the interaction between computers and human language. It enables computers to understand, interpret, and generate human language in a way that is both meaningful and contextually relevant. In this article, we explore the fundamentals of NLP and its profound impact on AI applications.
Understanding NLP: NLP involves a range of techniques and algorithms aimed at processing and analyzing natural language data. These techniques include tokenization, part-of-speech tagging, named entity recognition, syntactic parsing, semantic analysis, and sentiment analysis. By applying these techniques, NLP systems can extract valuable insights from text data and perform tasks such as language translation, text summarization, and question answering.
Impact on AI Applications: NLP has revolutionized various AI applications across different domains, enhancing human-computer interaction and enabling new capabilities. Some key areas where NLP has made a significant impact include:

- Virtual Assistants: NLP powers virtual assistants like Siri, Alexa, and Google Assistant, enabling users to interact with devices using natural language commands. These assistants can answer questions, perform tasks, and provide personalized recommendations based on user input.
- Language Translation: NLP has enabled breakthroughs in language translation, allowing for the automatic translation of text between different languages. Advanced NLP models like transformers have significantly improved translation accuracy, making cross-lingual communication more accessible and effective.
- Sentiment Analysis: NLP techniques are widely used for sentiment analysis, which involves analyzing text data to determine the sentiment or opinion expressed. Sentiment analysis has applications in social media monitoring, customer feedback analysis, and market research, helping businesses understand public opinion and sentiment towards their products or services.
- Text Generation: NLP models like recurrent neural networks (RNNs) and transformers have the ability to generate human-like text based on input prompts. These models are used for tasks such as text summarization, content generation, and dialogue systems, enabling AI systems to produce coherent and contextually relevant text.
- Information Extraction: NLP techniques such as named entity recognition and relation extraction are used for extracting structured information from unstructured text data. This information extraction process is valuable for tasks like information retrieval, knowledge graph construction, and data mining, facilitating the extraction of insights from large text corpora.
- Question Answering: NLP powers question answering systems that can understand and respond to natural language questions. These systems use techniques like semantic parsing and information retrieval to analyze questions and retrieve relevant answers from large text databases or knowledge bases.
Challenges and Future Directions: Despite its advancements, NLP still faces several challenges, including ambiguity, context understanding, and bias in language data. Addressing these challenges requires ongoing research and development in areas such as contextual understanding, multimodal NLP, and ethical AI.
Future directions in NLP research include improving language understanding capabilities through pre-trained language models, enhancing multilingual and cross-lingual capabilities, and developing AI systems that can understand and generate human-like conversations more effectively.
In conclusion, NLP plays a crucial role in advancing AI capabilities and enabling more natural and intuitive interactions between humans and computers. From virtual assistants and language translation to sentiment analysis and question answering, NLP has transformed various AI applications and continues to drive innovation in the field. As NLP research progresses, it holds the potential to further enhance human-computer interaction, enable new applications, and unlock the full potential of artificial intelligence.
More Articles
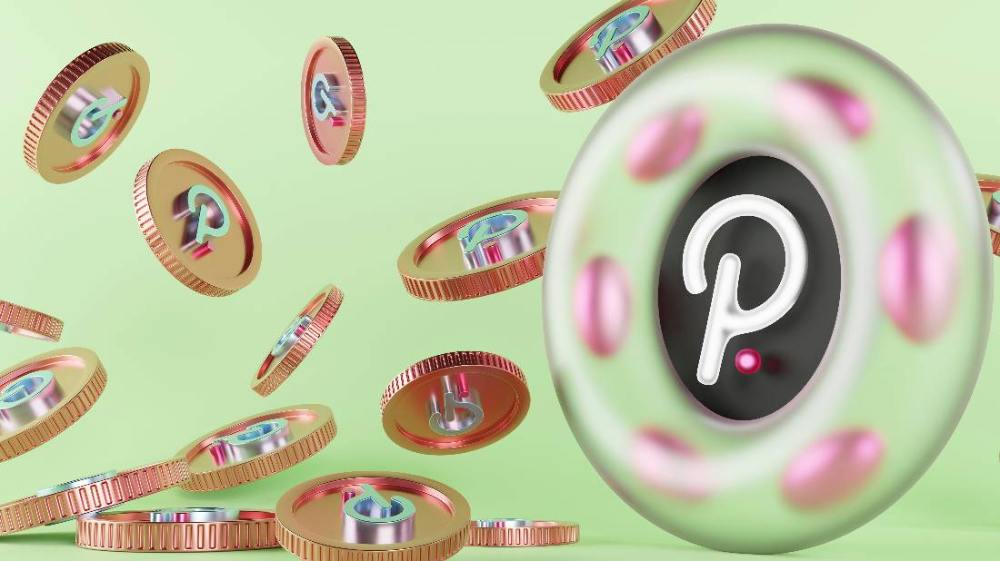
Blockchain Scalability Solutions: Overcoming Limitations for Mass Adoption
4 min read | 21 Jul 2024
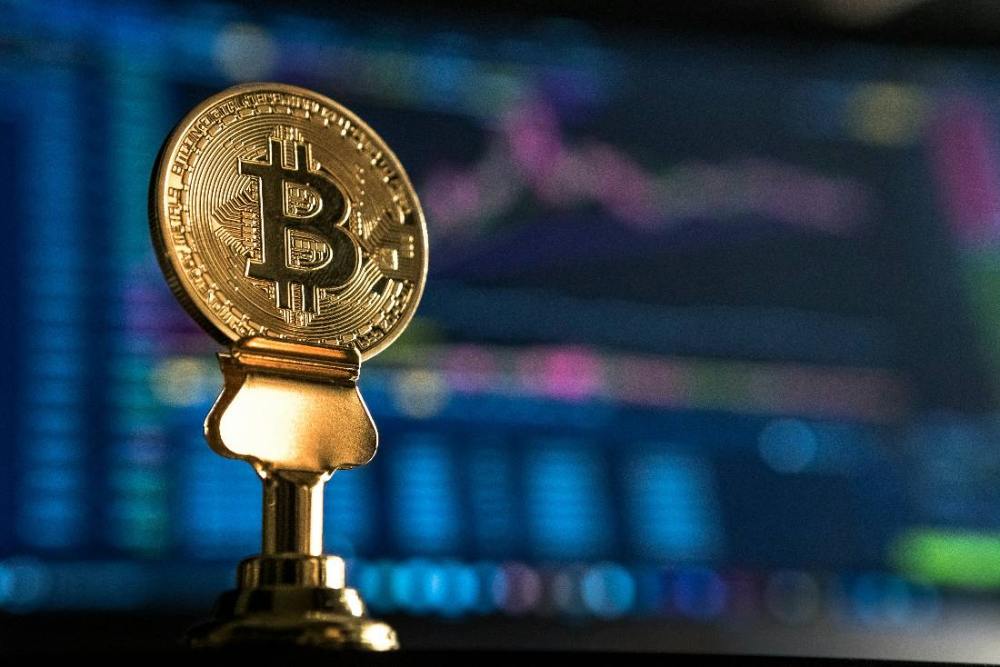
Privacy Coins: Enhancing Anonymity in Digital Transactions
5 min read | 20 Jul 2024
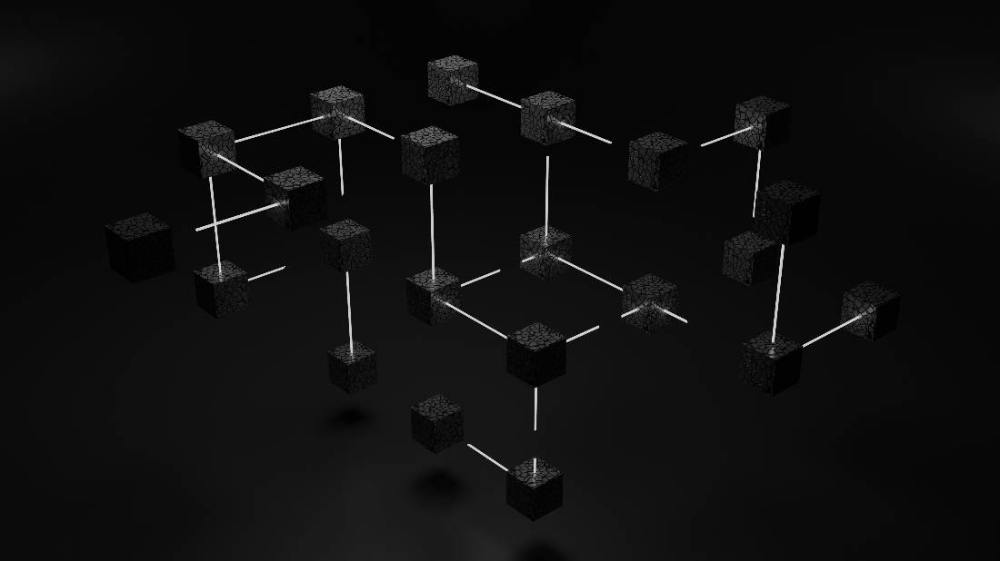
The Impact of Blockchain on Supply Chain Transparency
3 min read | 19 Jul 2024

Cryptocurrency Regulation: Balancing Innovation and Compliance
7 min read | 18 Jul 2024
More Articles

Permissioned vs. Permissionless Blockchains: Which is Better?
5 min read | 04 Jul 2024
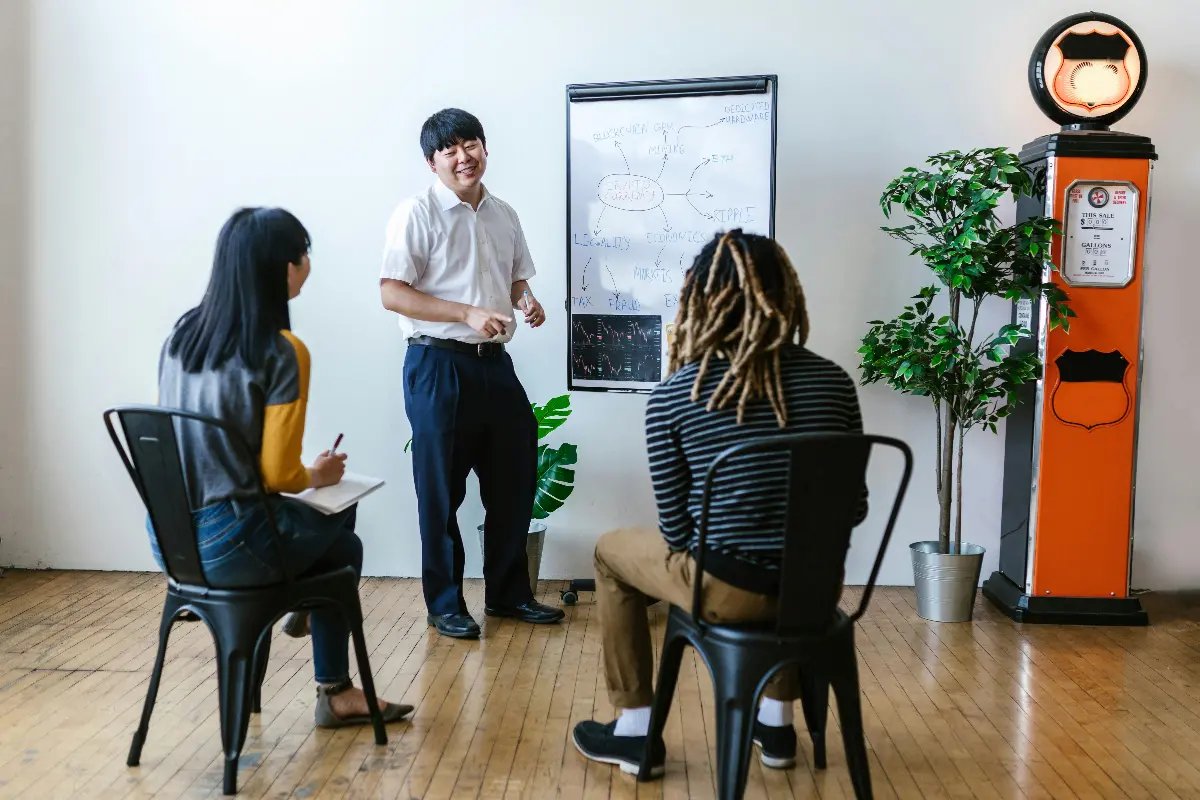
Nodes in Blockchain: The Building Blocks of Decentralization
3 min read | 03 Jul 2024
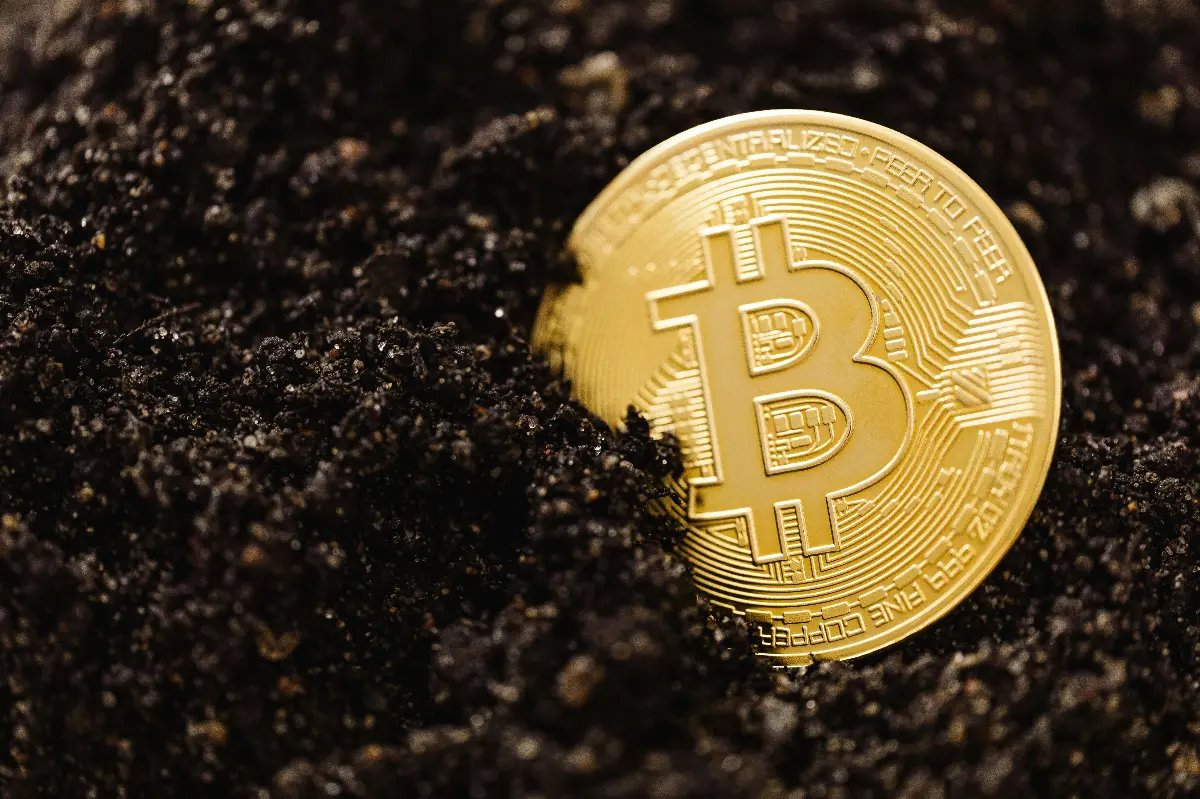
Blockchain Mining: The Backbone of Cryptocurrency Networks
5 min read | 02 Jul 2024
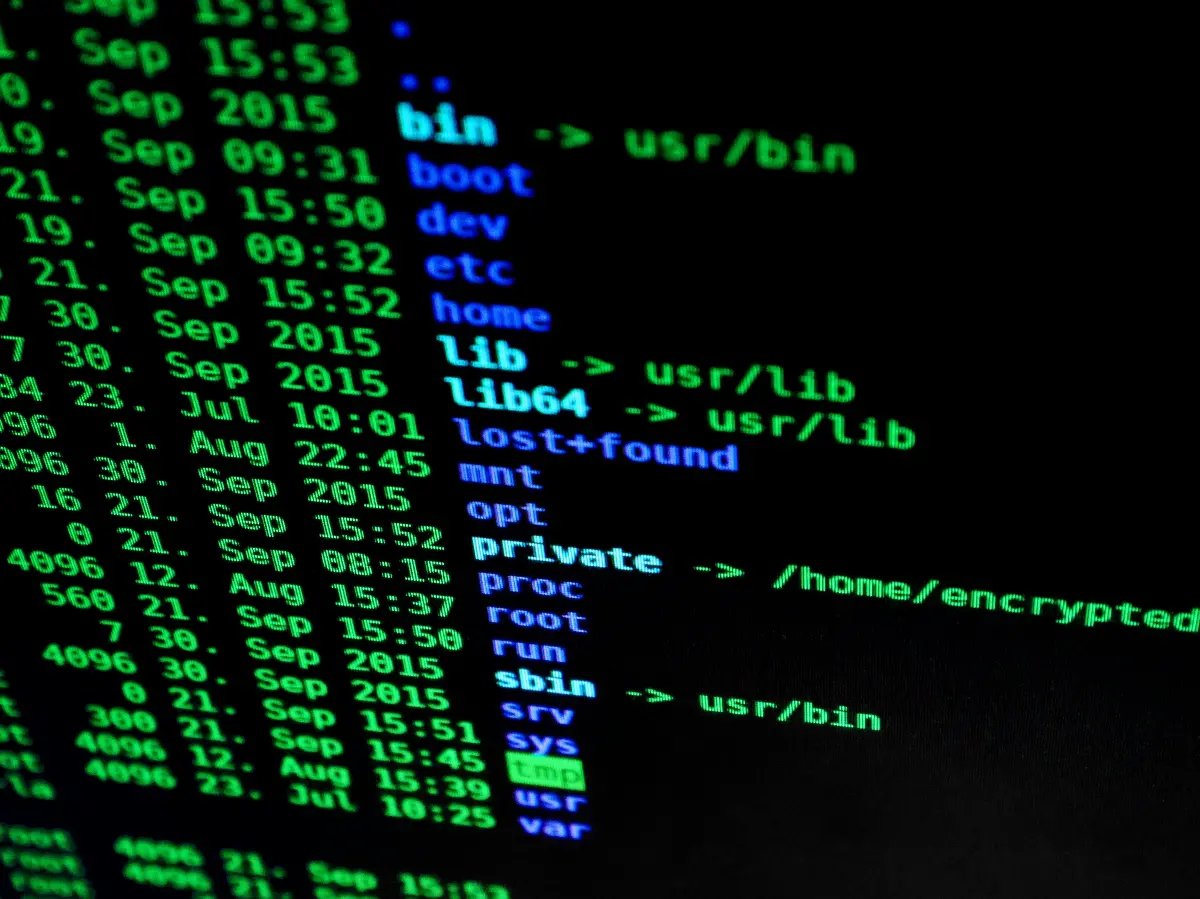
The Immutable Nature of Blockchain: Security and Data Integrity
5 min read | 01 Jul 2024